When you open TikTok, the For You Page (FYP) immediately starts serving content seemingly perfectly tailored to your interests. This personalized stream keeps billions of users scrolling for hours, making TikTok one of the most engaging platforms in social media history. But what’s happening behind the scenes? How does TikTok decide which videos, out of millions uploaded daily, deserve a spot on your FYP? Let’s decode the mysterious forces that power TikTok’s recommendation engine.
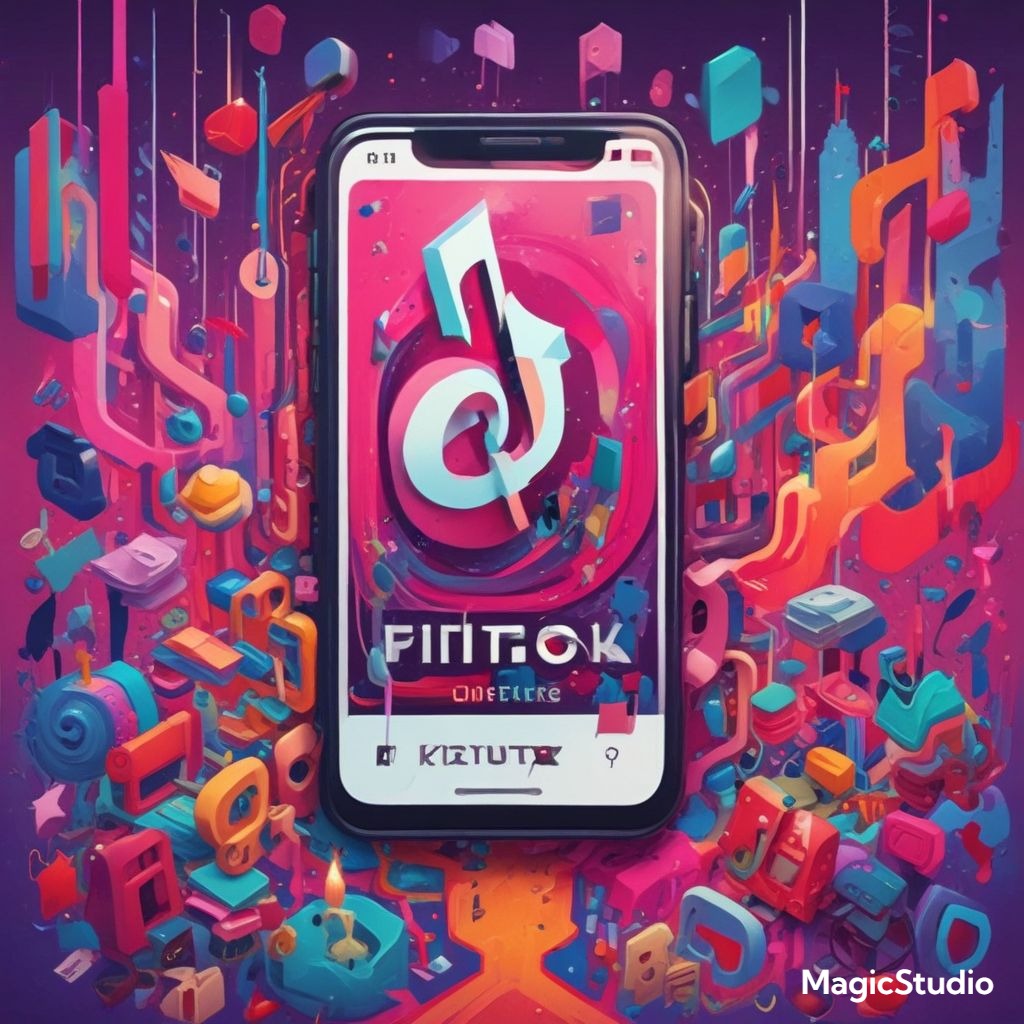
The Core Elements of TikTok’s Recommendation System
TikTok’s algorithm operates as a sophisticated recommendation system built on machine learning principles. Unlike platforms that rely heavily on your explicit social connections, TikTok prioritizes content affinity and engagement patterns.
The algorithm works continuously in real-time, analyzing every interaction you have with the platform. Each video you watch, like, share, or skip contributes data points that help the system understand your preferences with increasing precision. This creates a feedback loop where your actions directly influence future recommendations.
What makes TikTok’s approach unique is its willingness to test unproven content on small audience samples. This experimental approach enables the discovery of new creators in a way that more conservative algorithms might miss.
Social media researcher Dr. Maya Phillips explains: “TikTok’s innovation lies in its bold content distribution approach. Rather than waiting for content to prove itself through a creator’s established audience, it tests videos with small, strategically selected viewer groups to identify potential viral hits before they’ve gained traction.”
User Interactions: The Primary Signals
The strongest signals influencing TikTok’s algorithm come directly from how users interact with content. These interactions serve as explicit feedback that shapes the algorithm’s understanding of content quality and user preferences.
Watch time stands as perhaps the most powerful metric. When you watch a video completely—or even better, multiple times—you’re sending a clear signal that the content resonated with you. TikTok’s algorithm interprets full video views as strong endorsements, particularly for longer content where maintaining attention requires genuine interest.
Beyond simply watching, your active engagements significantly impact future recommendations. Comments carry particularly strong weight, as they require more effort than passive consumption or quick likes. The algorithm appears to interpret comments as evidence of deeper engagement, especially when they’re substantive rather than generic.
Sharing behavior sends powerful signals about content quality. When you send a TikTok to friends or share it on other platforms, the algorithm registers this as a strong endorsement. Videos generating high share rates typically receive broader distribution, as sharing indicates content compelling enough to personally recommend.
Content creator Sophia Martinez noticed this pattern across her viral videos: “Every TikTok that’s broken 100,000 views followed the same pattern—it started with higher-than-average share rates among my first few thousand viewers. Once people start sharing, the algorithm seems to accelerate distribution almost immediately.”
The “not interested” button and videos you quickly scroll past also provide valuable feedback. The algorithm learns as much from what you reject as what you embrace, continuously refining its understanding of your preferences through both positive and negative signals.
Content Characteristics That Matter
Beyond user interactions, the algorithm analyzes numerous content attributes to determine appropriate distribution and audience matching.
Captions, hashtags, and sounds provide contextual information that helps the algorithm categorize content. These elements help TikTok understand what your video is about and which interest communities might appreciate it. Strategic use of these elements increases the likelihood of reaching viewers with relevant interests.
Video features like effects, language, and aesthetic style contribute to categorization. The algorithm identifies patterns in visual elements to match content with users who have historically engaged with similar visual styles.
Trending elements receive temporary algorithmic boosts. Videos incorporating trending sounds, effects, or hashtags often receive wider initial testing, though this advantage diminishes if user engagement proves disappointing.
Content completion rate significantly impacts distribution. Videos that keep viewers watching until the end typically receive preferential distribution compared to content with high abandonment rates, even if other metrics appear strong.
Digital marketing strategist James Wilson observed: “After analyzing performance data across hundreds of TikTok videos, we’ve found that videos maintaining at least 80% average completion rates typically receive 3-4 times more algorithmic distribution than those with completion rates below 60%, even when initial engagement metrics look similar.”
Device and Account Settings
Technical and account-specific factors create another layer of algorithmic influence, though these typically exert more subtle effects than direct engagement signals.
Geographic location plays a significant role in content distribution. TikTok often prioritizes regionally-relevant content, particularly for users in non-English speaking regions or countries with distinct cultural contexts. This regional preference helps surface culturally relevant content while still allowing truly exceptional videos to cross geographic boundaries.
Language settings influence content matching, with the algorithm favoring content in your preferred language, particularly for text-heavy videos. However, visual-forward content frequently crosses language barriers when engagement signals are strong.
Device type and network conditions affect recommendation patterns in practical ways. Users on slower connections or older devices may receive more compressed content or shorter videos that align with their technical constraints.
Account age and usage patterns create subtle influences. New accounts undergo a more extensive “getting to know you” phase where the algorithm tests diverse content categories to establish baseline preferences. Meanwhile, power users who spend hours daily on the platform typically receive more experimental content than occasional users.
TikTok’s Content Categorization System
Behind TikTok’s seemingly magical content matching lies a sophisticated content categorization system. The platform uses machine learning to analyze and tag videos across hundreds of interest categories and subcategories.
This classification happens automatically as content is uploaded. TikTok’s visual recognition systems identify objects, settings, activities, and themes within videos. Audio analysis simultaneously categorizes sounds, music, speech patterns, and emotional tones. Together, these systems create a multi-dimensional content profile for each video.
These content profiles get matched against user interest profiles that the algorithm continuously updates based on engagement history. When strong matches occur between content attributes and user preferences, the video becomes a candidate for that user’s FYP.
Tech journalist Rebecca Chen explains: “TikTok essentially creates a multidimensional map of all content on the platform. Each video occupies a specific location in this conceptual space. Your personal preference profile occupies another location, and the algorithm serves videos that exist closest to your preference point in this mapped space.”
The Distribution Testing Process
TikTok employs a phased distribution approach that gives every video a chance at virality while quickly identifying the most engaging content.
When you post a new TikTok, the algorithm serves it to a small initial audience, typically a few hundred viewers. These viewers aren’t randomly selected but rather users who have engaged with similar content or your previous videos. This initial test group provides critical engagement data.
If your video generates above-average engagement from this test audience, the algorithm expands distribution to a second, larger wave of viewers. Each successful wave leads to broader distribution, creating potential for exponential reach. Videos failing to meet engagement thresholds at any stage see their distribution limited.
This iterative testing explains why view counts often come in distinct jumps rather than steady increases. Each jump typically represents the algorithm approving the video for a wider distribution circle after successful performance with previous audiences.
Content strategist Michael Torres describes this as “algorithmic gateways” that videos must pass through: “Each video essentially faces a series of performance reviews. Pass one review, and you reach more viewers. The genius of this approach is that truly engaging content can quickly reach massive audiences regardless of the creator’s follower count.”
The Interest Graph vs. Social Graph
Traditional social platforms like Facebook and Instagram rely heavily on social connections—who you follow and who follows you—to determine what content appears in your feed. TikTok takes a fundamentally different approach by prioritizing interest affinity over social connections.
This interest-based approach explains why TikTok can feel so addictively relevant despite showing content primarily from creators you don’t follow. The algorithm focuses on matching content to your preferences rather than limiting your feed to social connections.
While following accounts still influences your feed, TikTok maintains a heavy bias toward interest relevance over social connections. This interest-first approach enables the platform’s renowned content discovery capabilities and creates more equitable distribution opportunities for new creators.
Social media researcher Dr. Alex Rivera notes: “TikTok’s revolutionary contribution to social media design is deprioritizing the social graph in favor of the interest graph. By focusing primarily on content affinity rather than social connections, TikTok created a discovery engine that surfaces relevant content regardless of who created it.”
The Feedback Loop: How Your Actions Shape Your Feed
Each interaction on TikTok refines the algorithm’s understanding of your preferences, creating a continuously evolving personalized experience.
When you engage positively with certain content categories, the algorithm increases similar content in your feed. This reinforcement creates interest clusters in your recommendations. If you watch cooking videos consistently, you’ll see more culinary content, often with increasingly specific targeting based on engagement patterns.
Passive signals matter too. The algorithm tracks how long you pause on videos without engaging, which videos you rewatch, and even subtle signals like slowing your scroll before moving on. These signals help build a more nuanced understanding of your interests.
This adaptive system creates both opportunities and challenges for users. The responsive nature provides highly engaging content but can also create “filter bubbles” where you see increasingly narrow content types. Breaking out of these patterns requires deliberate exposure to diverse content.
Digital behavior specialist Priya Sharma explains: “TikTok’s algorithm responds dramatically to sustained changes in engagement patterns. If you consciously engage with different content for even 2-3 days, you can significantly reshape your feed. The algorithm rewards active curation through engagement much more than explicit controls.”
Time-Based Factors and Content Freshness
Recency plays a significant role in TikTok’s recommendation system, though with more nuance than many users realize.
New content receives temporary distribution advantages, as the algorithm needs fresh testing data to evaluate performance. This recency bias helps newer uploads get initial visibility but doesn’t guarantee sustained distribution without strong engagement.
Time-of-day patterns influence content matching. The algorithm appears to consider when users are most responsive to certain content types. Entertainment content may perform better in evening hours, while informational content might see stronger morning engagement. These patterns affect when your content gets its testing opportunities.
Seasonal relevance impacts distribution for topical content. Videos relating to current holidays, seasons, or cultural moments receive temporary relevance boosts during appropriate periods. This temporal awareness helps the algorithm surface timely content when contextually appropriate.
Video age impacts but doesn’t determine distribution potential. While newer content generally receives preferential initial testing, high-performing older videos can continue receiving substantial distribution for weeks or even months if engagement remains strong.
Content strategist Jennifer Park observed this longevity effect: “We’ve tracked videos that continue receiving significant algorithmic distribution 60-90 days after posting because they maintained exceptional engagement metrics. The algorithm seems to have a ‘quality override’ that keeps serving high-performing content regardless of age if users keep responding positively.”
Moderating Factors and Safety Controls
TikTok’s algorithm balances content optimization with safety and community standard enforcement, creating essential guardrails on pure engagement-based distribution.
Content review systems scan for policy violations before videos enter distribution. This automated screening looks for potential violations across categories like harmful content, copyright infringement, and misinformation. Content flagged during this process faces limited distribution or removal.
The algorithm incorporates diversity controls to prevent feed monotony. Even if you intensely engage with one content type, the system periodically introduces diverse content to prevent extreme narrowing of your recommendations and maintain discovery opportunities.
Age-appropriate content filtering creates different distribution patterns based on user age. Certain content categories face restricted distribution to younger users, even when engagement metrics would otherwise justify broader reach.
Viral velocity monitoring helps identify potentially problematic trending content. The algorithm includes systems that flag unusually rapid spread patterns for human review, particularly when combined with certain keywords or themes that correlate with past problematic content.
Social media policy expert Thomas Chen explains: “TikTok’s recommendation system actually contains multiple competing objectives. Pure engagement optimization would simply show whatever keeps people watching longest, but the implemented system balances engagement with safety, diversity, and community health considerations, sometimes at the cost of maximum engagement.”
How Creator Metrics Influence the Algorithm
While TikTok emphasizes content quality over creator status, certain creator-specific factors do influence algorithmic distribution.
Creator consistency affects how the algorithm treats new uploads. Accounts posting regularly typically receive more reliable initial testing for new content compared to sporadic posters. This consistency advantage appears strongest when posting frequency aligns with audience engagement patterns.
Account standing impacts distribution opportunities. Creators who’ve violated community guidelines or received multiple content removals often experience more conservative initial distribution for new uploads, requiring stronger performance metrics to achieve wider reach.
Creator response rates to comments may influence distribution. Accounts that actively engage with their comment sections appear to receive modest algorithmic advantages, as this engagement cultivates community interaction that benefits the platform ecosystem.
Social media analyst Jessica Morgan notes: “We’ve observed that accounts maintaining comment response rates above 10-15% see approximately 20% better average distribution for new content compared to similar creators who rarely engage with comments. The algorithm seems to reward creators who foster community conversation.”
Historical performance creates general expectations for new content. Creators with track records of high-engagement videos typically receive more generous initial testing for new uploads, though each video must ultimately prove itself through its own performance metrics.
Myths and Misconceptions About the Algorithm
As with any complex system, TikTok’s algorithm has generated numerous misconceptions that influence creator behavior—often counterproductively.
The “first 24 hours myth” suggests that a video’s performance is determined entirely within its first day. While early engagement certainly matters, the algorithm continuously evaluates content. Videos frequently “pop” days after posting when they find the right audience match through continued testing.
The “shadowban” explanation gets overused when content underperforms. While TikTok does limit distribution for policy violations, most perceived “shadowbans” simply reflect natural performance variation or content that failed to resonate with test audiences.
Follower count has less impact than many assume. While followers create an initial viewership advantage, the algorithm quickly expands or limits distribution based on actual engagement metrics rather than creator popularity.
Content strategist David Lee challenges common misconceptions: “After analyzing thousands of TikTok videos, we’ve found that approximately 40% of videos that eventually reached over 100,000 views had below-average performance in their first three hours. The algorithm gives content multiple opportunities to find its audience if it shows promise with even small viewer segments.”
Adapting to Algorithm Updates
Like all technology platforms, TikTok continuously refines its recommendation system, requiring creators to stay adaptable rather than relying on fixed strategies.
TikTok typically implements algorithm adjustments gradually rather than through sudden overhauls. These evolutionary changes often focus on specific content categories or engagement patterns rather than transforming the entire system at once.
The platform’s fundamental values remain relatively stable even as implementation details evolve. Content that genuinely engages viewers, maintains attention, and drives meaningful interaction continues performing well across algorithm iterations.
Testing smaller content variations helps identify changing algorithmic preferences. Creators who systematically experiment with different content attributes (length, style, posting times) can detect shifting patterns before major strategy adjustments become necessary.
Digital marketing expert Ryan Torres recommends adaptability: “The most successful TikTok creators we work with view algorithm adaptation as an ongoing process rather than a one-time learning curve. They consistently test content variables and analyze performance patterns, allowing them to detect and adapt to distribution changes often weeks before creators who follow rigid formulas.”
Ethical Considerations in Algorithmic Curation
TikTok’s powerful algorithm raises important questions about content discovery, attention economics, and information exposure that extend beyond purely technical considerations.
Filter bubble concerns apply particularly to TikTok’s highly personalized recommendations. The algorithm’s efficiency at serving content matching your existing interests can potentially limit exposure to diverse perspectives or topics outside your established preferences.
Attention optimization creates both entertainment value and potential concerns. The system’s remarkable ability to identify highly engaging content keeps users entertained but also contributes to extended usage sessions that might displace other activities.
Content creator pressures emerge from algorithmic incentives. The system’s rewards for high-engagement content can push creators toward more extreme, emotional, or sensationalized content approaches to capture attention in competitive niches.
Digital ethics researcher Dr. Samantha Winters contextualizes these concerns: “TikTok’s algorithm represents both the promise and challenge of modern recommendation systems. Its ability to connect creators with genuinely interested audiences creates unprecedented opportunities, but the same systems raise legitimate questions about information diversity, attention impacts, and creator incentives that users should consider thoughtfully.”
Balancing Algorithmic Understanding with Creative Quality
Understanding TikTok’s algorithm provides valuable insight, but technical optimization can never replace compelling creative content. The most successful creators find a balance between algorithmic awareness and authentic content development.
Technical knowledge should inform but not dictate creative decisions. Understanding how the algorithm evaluates content helps shape effective presentations, but chasing perceived algorithmic preferences at the expense of content quality typically backfires through reduced audience connection.
Engagement patterns reveal valuable audience insights beyond algorithmic considerations. Analyzing which content resonates with viewers provides crucial feedback about audience preferences and interests that should guide content strategy independent of distribution tactics.
The algorithm ultimately amplifies viewer preferences rather than imposing arbitrary standards. Creating content that genuinely resonates with human viewers naturally aligns with algorithmic success, as the system fundamentally aims to identify content that real people find valuable.
Content strategist Michelle Zhang emphasizes this balance: “The creators who maintain sustainable TikTok success typically spend about 80% of their energy on creating genuinely valuable content and 20% on understanding platform mechanics. Those who reverse this ratio often achieve short-term views but struggle with audience loyalty and long-term growth.”
Practical Applications: Working With the Algorithm
For creators and brands looking to succeed on TikTok, certain practical approaches can help work effectively within the platform’s recommendation system.
Hook viewers immediately. The first 2-3 seconds determine whether viewers continue watching or scroll away. Strong openings dramatically impact completion rates, which directly influences further distribution.
Create for specific viewer segments rather than general audiences. The algorithm excels at finding the right niche audience for highly specific content but struggles to identify suitable viewers for broadly targeted, generic content.
Optimize for completion rates by matching content length to value delivery. Shorter videos should provide immediate value, while longer content needs sustained interest drivers throughout. Video length itself matters less than maintaining viewer attention throughout the duration you choose.
Encourage meaningful interaction through genuine prompts. Comments, shares, and other engagement actions significantly impact distribution, but forced or artificial calls-to-action often reduce overall engagement quality.
Social media strategist James Wilson advises: “Consider the algorithm your distribution partner rather than a target to manipulate. When you create content specifically designed to provide value to certain viewer types, the algorithm becomes remarkably effective at finding those viewers. The most successful approach focuses on genuine audience connection, allowing the algorithm to do what it does best—match your content with people likely to appreciate it.”
The Future of TikTok’s Recommendation System
TikTok’s algorithm continues evolving, with several development directions likely to shape its future operation.
Creator tools for algorithmic insights are expanding gradually. TikTok has introduced more detailed analytics about audience sources and engagement patterns, a trend likely to continue as the platform helps creators better understand performance factors.
Content categorization systems grow increasingly sophisticated. The platform continues refining its ability to understand nuanced content themes, particularly for specialized interest categories and emerging trends.
User control features have increased over time. TikTok has added more options for users to shape their feed preferences directly, likely responding to both user feedback and regulatory attention regarding algorithmic transparency.
Multimodal understanding represents a frontier for development. The platform continues advancing systems that simultaneously analyze visual elements, audio components, text overlays, and engagement patterns to create increasingly nuanced content matching.
Technology analyst Maria Chen predicts: “TikTok’s algorithm development appears focused on increasing precision rather than fundamentally changing approach. We’re likely to see more granular interest matching, better content understanding, and perhaps more explicit user controls, but the core system of testing-based distribution and engagement-driven amplification will likely remain the platform’s foundation.”
Conclusion: The Human Element in Algorithmic Success
Despite its technical sophistication, TikTok’s algorithm fundamentally serves as a matchmaking system connecting human creators with human viewers. Understanding its operation provides valuable context, but authentic connection remains the core driver of successful content.
The algorithm ultimately identifies and amplifies content that resonates with real people. It measures and responds to genuine human interest, meaning the most reliable path to algorithmic success involves creating content that truly matters to specific audiences.
This human-centered reality means that while the technical details of TikTok’s recommendation system will continue evolving, its fundamental purpose remains connecting people with content they find meaningful. Creators who focus primarily on authentic connection while maintaining awareness of platform mechanics position themselves for sustained success regardless of specific algorithmic adjustments.
Digital media researcher Dr. Jonathan Lee offers this perspective: “TikTok’s recommendation system succeeds precisely because it centers human response rather than arbitrary metrics. Creators obsessing over algorithm ‘hacks’ often miss that the system fundamentally measures whether real people find their content valuable. The most effective strategy isn’t tricking an algorithm—it’s connecting with actual people through creative work that genuinely resonates with them.”
In the end, while TikTok’s algorithm determines what appears on your For You Page through sophisticated technical systems, its decisions reflect a simple reality: it shows you what other humans like you have found engaging, meaningful, or valuable. This human foundation remains the constant amid evolving technical implementation, creating opportunities for creators who prioritize authentic connection within the framework of platform understanding.